Visual Analytics
Brainspace is a powerful yet practical artificial intelligence solution that enables you to make smarter, faster, and more informed data decisions. Our software provides a unique and patented approach to data analysis. Brainspace dramatically increases the speed and efficiency by which you analyze data across different use cases. Whether you’re performing an internal investigation or mining your organization’s data for actionable intelligence, Brainspace can improve your team’s efficiency by as much as 90%. The intuitive user interface can easily guide you down a path of uninterrupted data discovery. Several key benefits include:
The Power of Accelerated Decision Making
- Seamlessly connected data analytics features built within an easy-to-use interface.
- Point and click decision making power with interactive data visualizations.
- Automatically connects topics and people while surfacing unknown connections.
- Data Analytics tool purpose built for unstructured content.
- Supervised learning workflows that automate the machine learning process.
The Power of the 360 View
Brainspace enables analysts to apply multidimensional analysis, including temporal, conceptual, and social networking across the entire data set, helping them quickly uncover hidden connections and trends buried deep within an ever-growing mountain of data. Powerful text analytics highlight critical details masked in unstructured data such as email communication and surveillance reports.
Explore, Focus, & Confirm
Cluster Wheel
This interactive clustering visualization allows you to quickly explore topics of interest regardless of data size. Zoom in on topics of interest and easily isolate the signal from the noise in your data.
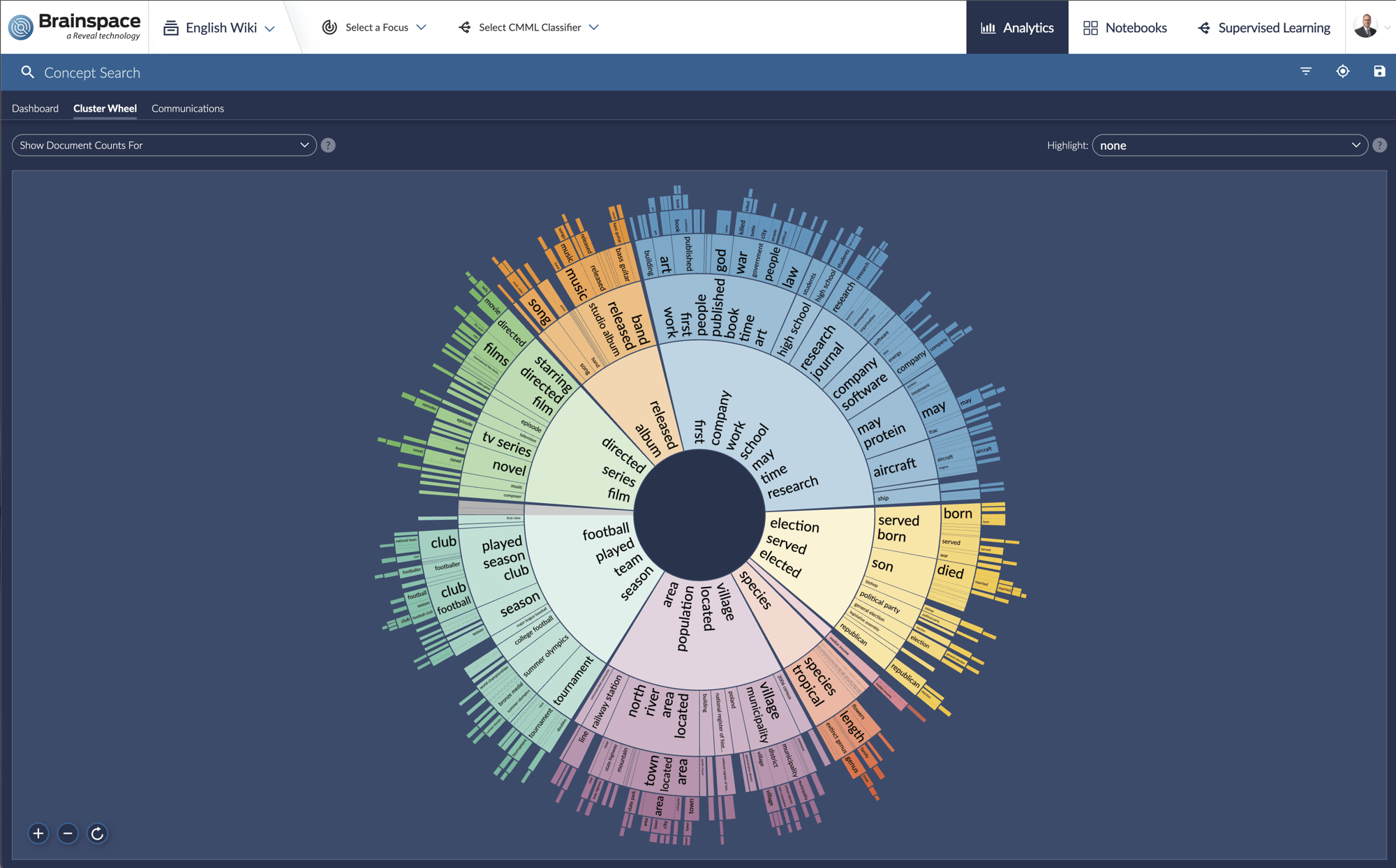
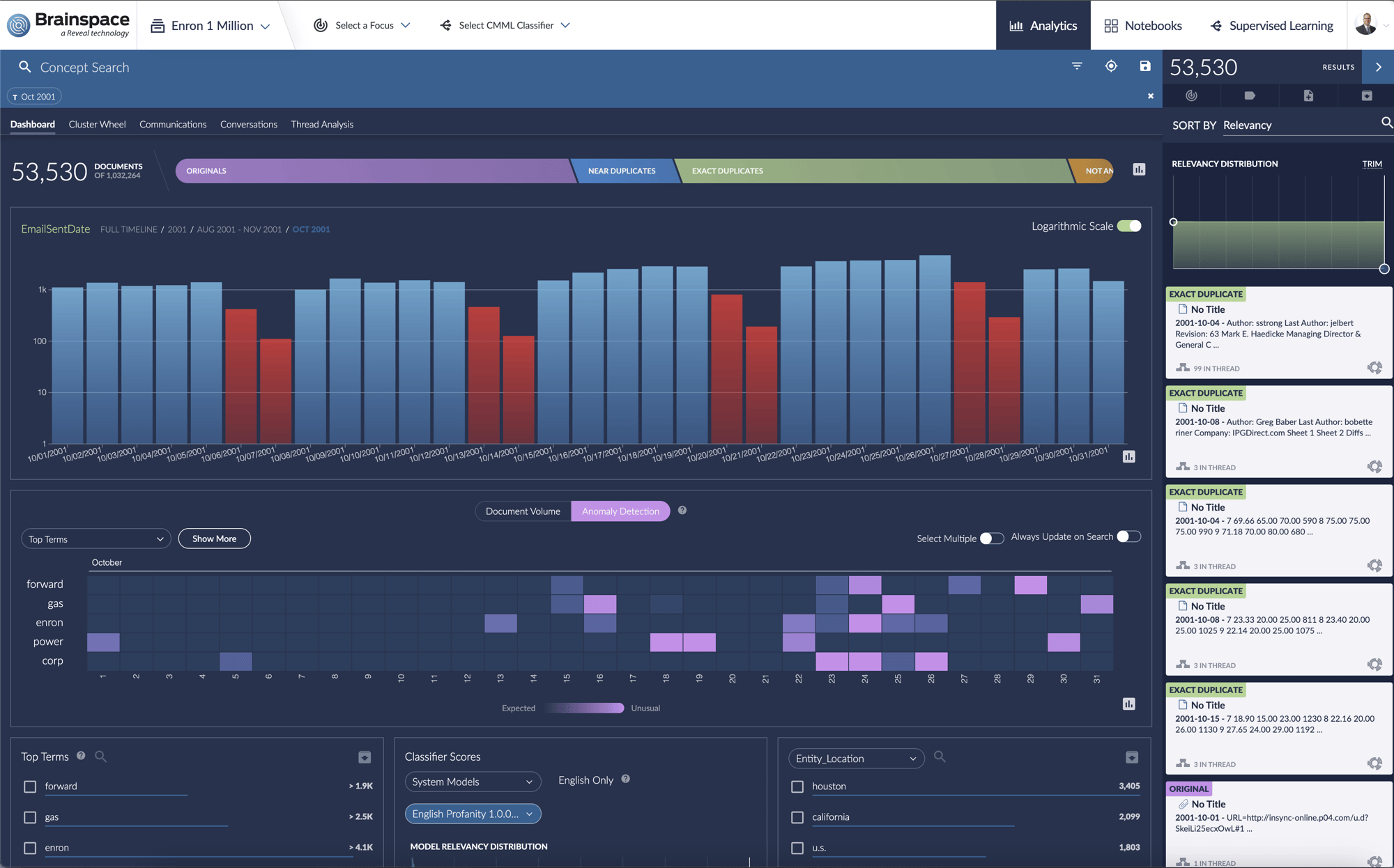
Point-and-Click Filtering
Meta Dashboard
This flexible graphic interface gives you an at-a-glance view of key search terms – and makes it easy to dig deeper. Point and click to see how term usage has varied over time, or use our term heat map to easily grasp connections between terms. You can zero in on relevant data about specific people, places and things with a filter on extracted entities, and customize your search to focus on individual people or domains.
Observe Communication Patterns
Communication Analysis
Your organization can quickly gain insight into communication patterns within the data, by mapping out who’s communicating with whom about topics that are important to you.
Communications analysis displays complete networks of communication and can be easily adapted to explore facets and interactive timelines. Users can quickly identify persons of interest and explore related people and conversations. Organizations can also gain insight into social media chatter and trending topics through this tool.

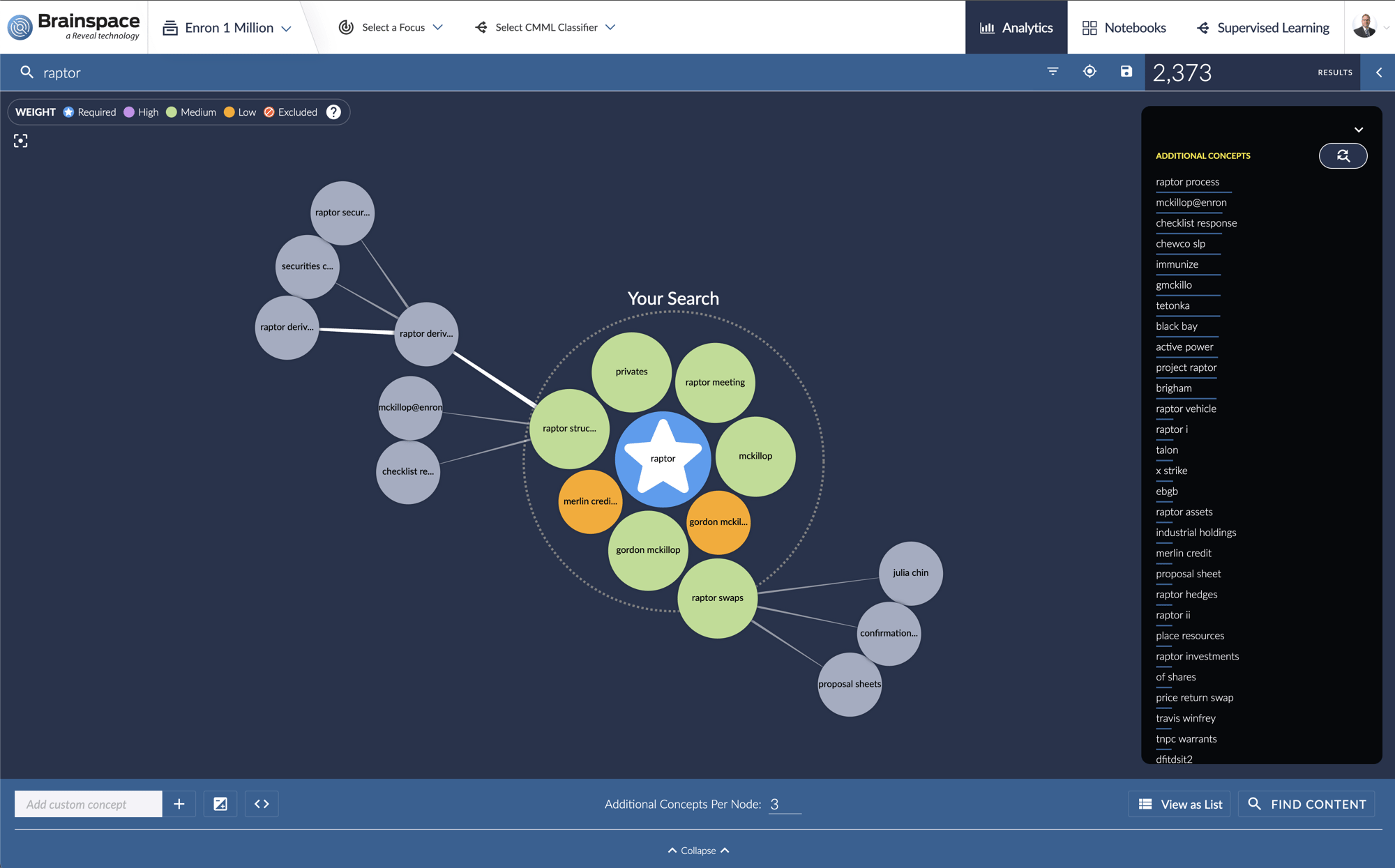
Search, Refine, & Reveal Hidden Connections
Transparent Concept Search
Sometimes the most relevant data isn’t exactly what you think it will be.
Transparent concept search lets you start with a phrase, a paragraph, or even entire document, then expand your search automatically to reveal related concepts. Often this tool can uncover key concepts that your organization wasn’t previously tracking.
Accelerate Speed to Relevance
Supervised Machine Learning
Your organization can quickly gain insight into communication patterns within the data, by mapping out who’s communicating with whom about topics that are important to you.
Communications analysis displays complete networks of communication and can be easily adapted to explore facets and interactive timelines. Users can quickly identify persons of interest and explore related people and conversations. Organizations can also gain insight into social media chatter and trending topics through this tool.
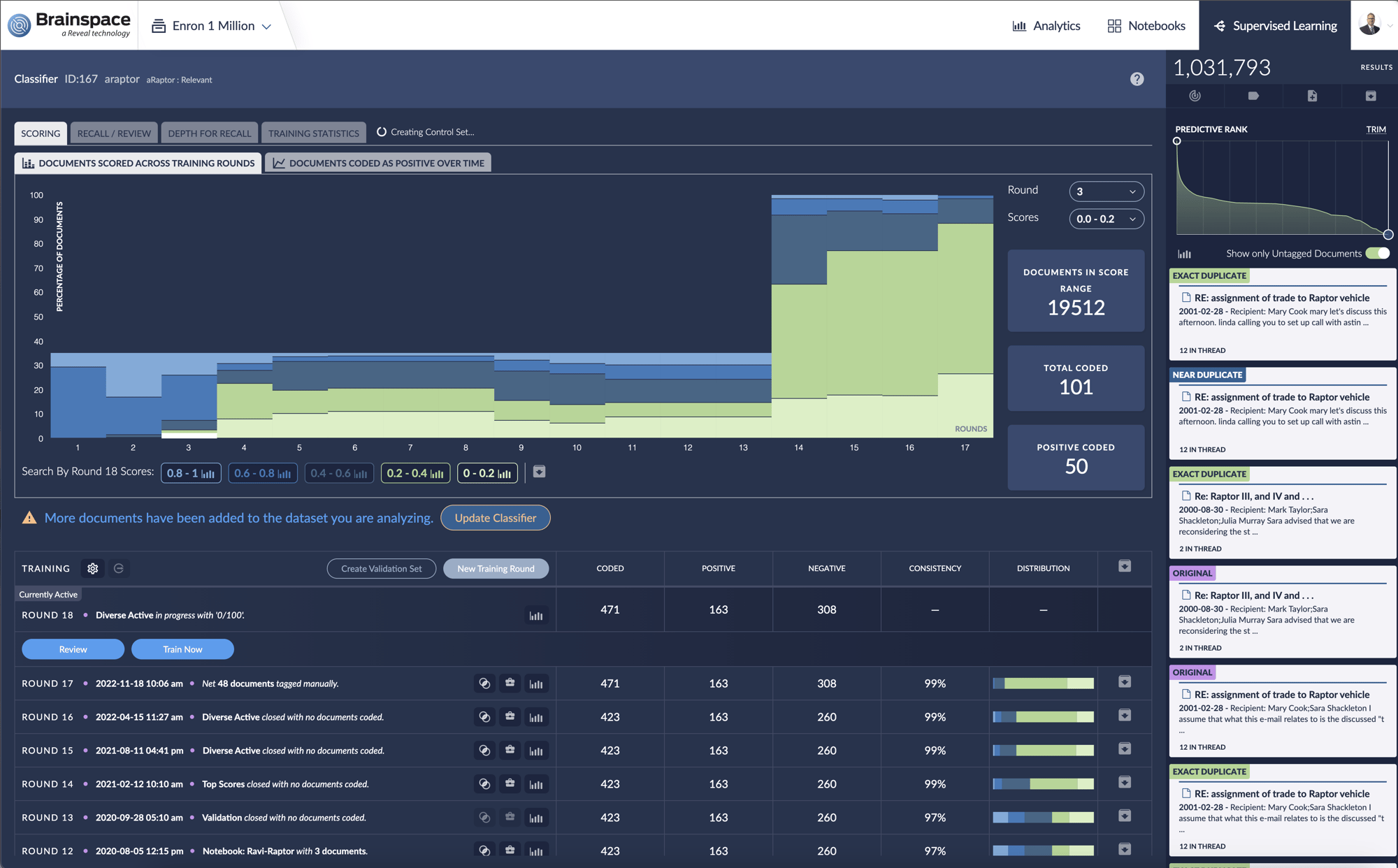
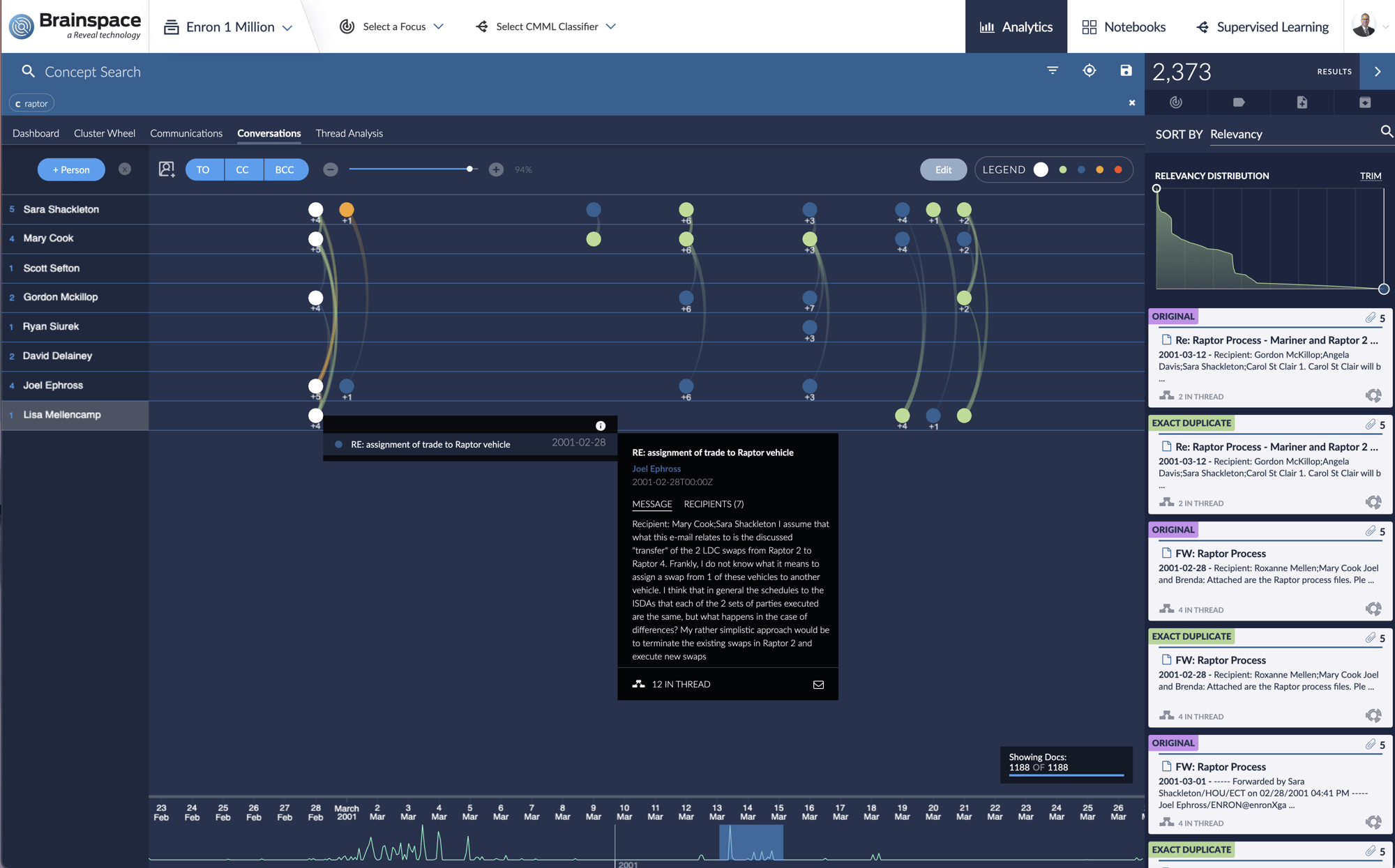
Identify Level of Participation within Specific Conversation Topics
Conversations
Understand how conversations unfold over time between selected participants. Easily pinpoint when sensitive information has been communicated. Determine whether external parties were introduced into the conversation. Allows you to easily identify who knew what and when.
Request a Demo
Continuous Multimodal Learning (CMML)
AI tools will never substitute for sound judgment and subject matter expertise, but they can automate, extend and apply your team’s knowledge to a wider array of documents. Continuous Multimodal Learning (CMML) simplifies the process of training a machine to find specific topics or events. Our software captures the way your team makes decisions about data using a range of text analytics tools, then applies next generation supervised learning to continually refine and improve results.

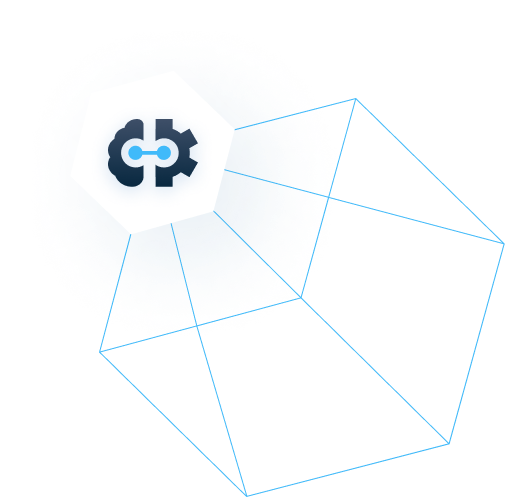
Portable AI Models
Once you’ve taught our software to find what you need, you can easily transfer those predictive models to new databases – allowing your organization to generate results faster than before.
Related Resources
Reveal is your trusted resource for optimized processes, best practices and trending technology. Here are a few resources on the topic of processing and early case assessment you may find useful.
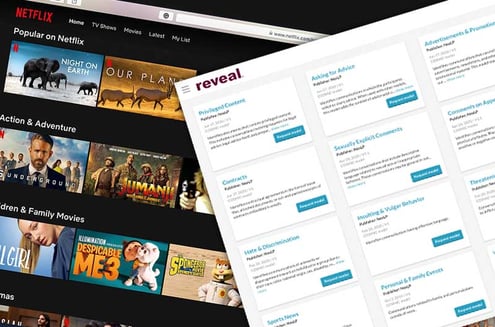
Like Netflix for Legal AI Models: Reveal’s AI Model Marketplace Goes Deep
What is the genius of Netflix? Is it that they have great TV shows and movies? No, paid providers...
Read Story